TOXIC FLOOD MODELING
Flood and chemical transport modeling
When chemical facilities experience rain and flooding during storms, toxic chemicals can be carried via stormwater (runoff), eroded soil, and flood waters caused by overflowing rivers and coastal storm surge that is driven by strong winds and sea-level rise.
In a novel approach, we coupled two water models: the watershed-scale Soil and Water Assessment Tool (SWAT) and the coastal Delft3D flood model, to characterize flooding caused by the combined effects of stormwater and storm surge, as well as how climate change may amplify storms and flooding in the future. The modeling results show where and how stormwater and flooding may affect facilities and move contaminants into vulnerable communities and ecosystems.
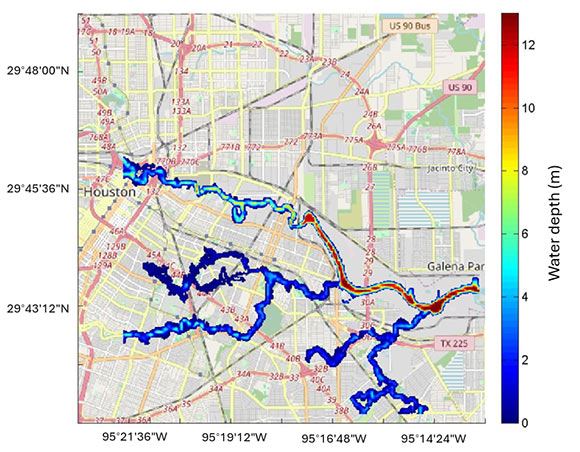
We used model results to generate a variety of indicators for the toxic flooding vulnerability assessment. At the facility level, the model provided information on flood and chemical transport characteristics including flood depth and duration based on historic and future weather conditions and potential chemical amount and concentration transported. At the community level, model results were incorporated into indicators for chemical transport potential, flood severity, and facility impacts. The model provided a physical basis for determining potential transport pathways between impacted communities and the combined contamination from multiple facilities. We weighted facility vulnerability scores by the model estimates of the potential contaminant transport to create indicators for combined facility impacts. Model-based indicators are all available to explore in our Vulnerability Map.
SWAT provided estimates of chemical transport in stormwater, eroded soil, and rivers, accounting for drainage from the entire Galveston Bay watershed, as boundary conditions and inputs into the Delft3D coastal model used to estimate flooding.
Learn more about the models below.
The primary physical effects to be captured that impact pollutant transport are inland flooding due to rainfall and swollen waterways, and surge inundation caused by hurricane winds. These hazard-related phenomena are further affected by tides and ocean waves. Tide levels can increase surge inundation by adding to the overall water level, while breaking waves on the coastline can add as much as 15% to surge water levels through the phenomenon of “wave set-up.” Both of these ancillary effects can modulate the severity of surge inundation.
The Delft3D model1 (also known as “Delft3D-FLOW”) used in this work was developed by Deltares, a nonprofit water research institute based in the Netherlands. Delft3D has been used in several studies in the Houston area2,3. The primary module of the model system is FLOW, a dynamic shallow water hydrodynamic model capable of simulating currents, water levels, and coastal inundation due to waves, winds, tides, and riverine flow. The model can be operated as a fully three-dimensional model or as a vertically-averaged two-dimensional model. We used the latter option, a standard choice for modeling of hurricane impacts. The model uses structured grids, and for this purpose we used a structured spherical (latitude-longitude) series of grids, with smaller, higher resolution grids receiving information from lower-resolution, larger grids, in a process known as “model nesting.”
The FLOW model requires inputs and other modules for forcing. The WAVE model, a module of Delft3D, was used to simulate the growth, propagation, and breaking of ocean surface waves. The WAVE model describes ocean waves in terms of their energy, and is a statistical representation of waves over time.
[1] Lesser, G.R., Roelvink, J.A., van Kester, J.A.T.M., and Stelling, G.S., “Development and validation of a three-dimensional morphological model.” Coastal Engineering, v 51., no. 8-9, p. 883, Oct. 2004.
[2] Zhu, R., Newman, G., Han, S., Kaihatu, J., and Wang, T., “An adaptive toolkit for projecting the impact of green infrastructure provisions on stormwater runoff and pollutant load – a case study on the city of Galena Park, Texas, USA.” Landscape Architecture Frontiers, vol. 11, no. 2, p. 72, Nov. 2023.
[3] Han, S., and Kaihatu, J.M., “Variability in surge levels in communities adjacent to the Houston Ship Channel Industrial Corridor to changes in hurricane characteristics.” Coastal Engineering Journal, doi: 10.1080/21664250.2023.22903330, Feb. 2024.
The Delft3D-FLOW model does not have a fate and transport model for industrial hazards. As a workaround, we used numerical “drogues” to track the transport of potentially-contaminated water masses in the model. These drogues are tagged numerical particles whose positions are tracked over the course of the simulation. This offers insight into the extent of contaminant transport; however, no transformation or dilution of the contaminant in flood waters is modeled.
Drogues were placed in the model at the location of facilities within each domain at the start of the simulation. The drogues remained stationary until flood waters from surge and/or riverine flooding reached them. In addition to determining the overall extent of possible contaminant transport from each facility, the drogues would also collect within topographic depressions, showing locations most at risk from contaminated floodwater.
The Delft3D-FLOW simulations comprised several domains. Winds over the entire Gulf of Mexico, and estimated tidal elevations across the Florida and Yucatan Straits, were used to generate waves and tidal elevations over the Gulf in the model.
The overall gridded domain consisted of a series of six levels of grids, progressing toward smaller domains of higher spatial resolution. The first five levels brought waves, currents, and water levels from the Gulf of Mexico, over the Texas continental shelf and through Galveston Bay, and into the Houston Ship Channel and Buffalo Bayou region. The grid resolutions varied from 10 km for the Gulf of Mexico grid, to between 30 m to 100 m for the various gridded domains at the final grid level. The exact resolution at the final level depended on the presence of small waterways and other finescale geographic features within the domain.
At the final level, six different subdomain grids were used to capture flooding and surge events within six different communities and immediate environs: Galena Park, Texas City, Channelview, Mont Belvieu, Laporte, and Sheldon. These six areas were selected based on the density of industry present within each, as well as flood history.
Flooding within three different time periods was simulated with the Delft3D model. During 2015, these areas experienced a relatively wet spring, while Hurricane Harvey occurred during 2017; thus these two years were simulated in the model. To represent future climate change conditions, input riverine flow from the SWAT model was increased by 7% for the time of Hurricane Harvey; the associated 2017 winds and tides were not correspondingly altered. The simulation for this climate change condition was limited to be between May 15 and October 15, rather than the entire year.
The computational time step varied depending on the spatial resolution and the CFL numerical stability criterion. For the six innermost nested spatial domains,, this time step varied between 6 seconds to 15 seconds.
Spatial maps of the water levels inside each of the six domains were output either hourly (for areas with relatively dynamic flooding) or once every two hours. Drogue positions were output at the computational time step of the simulation.
Data on the water depth (bathymetry) and land elevation (topography) were obtained from the National Center for Environmental Information (NCEI) via the Delft Dashboard model setup software. The NCEI database contains various topographical and bathymetric databases which were melded to create the terrain data for the model. For the greater Gulf of Mexico region, the General Bathymetric Chart of the Oceans (GEBCO) data set was used. This global data set is comprised of water depth data at a resolution of 15 arc-seconds (roughly 450 meters). Near to the coast, the Coastal Relief Model was used for the bathymetry and topography. This data has 1 arc-second resolution (about 30 meters). Finally, the Continuously Updated Digital Elevation Model (CUDEM) database for Texas was used where it was available. This data set has a resolution of 1/9 arc seconds (about 3 m), and was a valuable source of information for resolving the geography of creeks and waterways, as well as the Houston Ship Channel.
Winds for the year-long simulations were obtained from the Climate Forecast System Reanalysis (CFSR) database maintained by the National Center for Environmental Prediction (NCEP), NOAA. This database is comprised of a combination of modeled wind fields and satellite observations. The horizontal resolution of the data is approximately 0.5 degree latitude and longitude (about 55 km north to south and about 48 km east to west), with a one-hour temporal resolution.
For rapidly varying winds, such as those of a hurricane, the spatial resolution of the CFSR windfield may not be sufficient. This is particularly true for the Hurricane Ike case study, since the hazard for this event was primarily wind driven surge. In this event, we used the HURDAT2 database from the National Hurricane Center (NHC) for hurricane winds. This is a reduced data set which provides general parameters regarding the hurricane (radius to maximum winds, central pressure, latitude and longitude of the center along the hurricane track), as well as the distance from the center to the location of the 34 kt (17.5 m/s); 50 kt (26 m/s) and 64 kt (33 m/s) wind contours along the eight compass directions (N, S, E, W, NW, NE, SW, and SE). These are provided once every six hours, starting from when the storm is first recognized by the NHC to when the center ceases tracking it. The parameterization of Holland1 was used to fill in the wind field from these parameters.
Time series of tidal elevations were provided along the grid boundaries bisecting the Florida and Yucatan Straits, comprising the southeast corner of the Gulf of Mexico grid. The time series were provided by the Oregon State Inverse Tidal Model2, which assimilates satellite observations of sea surface height into a tidal model for accurate tidal elevations. The tidal elevations along these boundaries were then allowed to propagate into the Gulf of Mexico.
Riverine and rainfall-induced flooding were provided by the SWAT model. This input was in the form of daily-averaged discharge inflows to each of the six subdomains at locations corresponding to the boundaries of the SWAT subdomains that occur inside the six Delft3D subdomains.
All Delft3D model inputs, with the exception of CFSR winds and SWAT discharges, were accessed from the various databases by the Delft Dashboard model setup software.
[1] Holland, G., “An analytic model of the wind and pressure profiles in hurricanes.” Monthly Weather Review, v. 136, p. 1212.
[2] Egbert, G., and Erofeeva, S., “Efficient inverse modeling of barotropic ocean tides.” Journal of Atmospheric and Oceanic Technology, v. 19, p. 183, Feb. 2002
Outputs from the model consisted of wave heights, water levels, water depths, depth-averaged velocities, and other ancillary flow and wave information. These were provided at the spatial resolution of the model grid, and at a temporal resolution specified in the model input (either one or two hours). In addition, locations of drogues were also output by the model. Additional post-processing was required to make the output useful for inclusion in the vulnerability assessment as facility and community level vulnerability indicators.
Spatial maps of water depths (water level above datum minus the local land elevation) were output by the model at the pre-set time intervals. Ensuing analysis required a metric for water depth that typified an entire year or event, so the output was analyzed to determine the maximum water depth at every grid cell over the course of a year or event. It was also necessary to eliminate cells which were filled with water under normal conditions (e.g., portions of the Ship Channel or Buffalo Bayou, rivers) from the maximum flood depth calculation. Since it requires some time for the discharge from SWAT to propagate through the Delft3D subdomain along the watercourses, each subdomain was inspected to determine the point in time when an equilibrium in water depth appeared to be reached. Areas filled with water at that time were assigned NaN (not a number) and thus eliminated as a cell for which maximum flood elevation was calculated.
Another important flood characterization parameter is residence time – the length of time that flood water remains in an area. Since it is difficult for the model to go to zero water depth after flooding, we instead looked at the slope of the water depth with time. A positive slope (increasing water depth) indicates that the area is filling with water, while a negative slope (decreasing water depth) denotes drainage out of the area. For any given cell, we tracked the time from the point where the water depth slope over time went from positive, to the point where a negative slope was quickly followed by a slightly positive slope or a zero slope. The difference between those times was used as the residence time for that specific flooding event in that grid cell, which may have been one of several over the course of a year. The maximum, minimum, and average retention time over the course of a year or event was then determined for each cell.
Drogues were placed in the model at the locations of facilities, at the beginning of the simulation. These locations are always dry until reached by flood waters. The specific scenario replicated would be the case of a spill occurring prior to flooding, with flood water then transporting the spilled material through the domain.
Most information from the model and used in the vulnerability assessment and other tools were processed using MATLAB codes developed within the project. Translation between the native NEutral FIle System (NEFIS) format (developed by Deltares) and MATLAB binary formats was performed using MATLAB routines developed by Deltares. In-house MATLAB codes were then developed to perform the required analyses from the model results. Maps of residence time and maximum flood depths were output in TIFF format. Drogue tracks were written into ASCII files identified by facility ID. Each drogue file consisted of three columns: time (in minutes) from the start time of the simulation, and latitude and longitude of the drogue.
A number of watershed hydrologic models are available for water quality and quantity analysis with various strengths and weaknesses. We selected the Soil and Water Assessment Tool (SWAT), developed by researchers at Texas A&M University and USDA ARS, to provide plausible freshwater, sediment and contaminant flows under different weather scenarios as inputs to the hydrodynamic coastal model; and to locate vulnerable source areas and downstream impacted areas as targets for nature-based mitigation strategies.
SWAT is a widely-used semi-distributed watershed hydrology model, freely available1 and actively supported by a global community of modelers and developers. SWAT has been validated in the prediction of flashflood discharge 2 and has been applied to the Galveston Bay region for estimation of fresh water and sediment inflows3,4and the impact of precipitation and land use changes on nearby Mission-Aransas National Estuarine Research Reserve5 SWAT physical processes include: a rainfall/runoff curve number-based approach to surface hydrology, vertical and lateral groundwater transport and storage, reservoir dynamics, eroded soil and sediment processes, chemical fate and transport dynamics including partitioning, transformation, and transport in different media (soil, runoff, surface water, sediment), and land use management. Complete theoretical documentation may be found at: https://swat.tamu.edu/docs/.
[1] “SWAT | Soil & Water Assessment Tool.” [Online]. Available: https://swat.tamu.edu/. [Accessed: 24-Jul-2019].
[2] L. Boithias, S. Sauvage, A. Lenica, H. Roux, K. Abbaspour, K. Larnier, D. Dartus, and J. Sánchez-Pérez,“Simulating Flash Floods at Hourly Time-Step Using the SWAT Model,” Water, vol. 9, no. 12, p. 929, Nov.2017.
[3] T. Lee, R. Srinivasan, J. Moon, and N. Omani, “Technical Note: Estimation of Fresh Water Inflow to Bays from Gaged and Ungaged Watersheds,” Applied Engineering in Agriculture, vol. 27, no. 6, pp. 917–923, 2011.
[4] N. Omani, R. Srinivasan, and T. Lee, Estimating Sediment and Nutrient loads of Texas Coastal Watersheds with SWAT, vol. 4. 2012.
[5] C. R. Castillo, İ. Güneralp, and B. Güneralp, “Influence of changes in developed land and precipitation on hydrology of a coastal Texas watershed,” Applied Geography, vol. 47, pp. 154–167, Feb. 2014.
In our study, we were concerned about chemicals that are persistent – present in the environment long enough to expose humans and ecosystems; mobile – readily transported from their industrial petrochemical sources in flood waters and eroded soil and sediment that are caused by stormwater and storm surge; and toxic – harm the health of humans, plants and animals who come into contact with them. Specific chemical classes of concern that carry these traits include: polycyclic aromatic hydrocarbons (PAHs), per- and polyfluoroalkyl substances (PFAS), and metals.
Given this focus, we modeled chemical transport in SWAT for generalized chemical classes, assuming contaminants had negligible transformation/biodegradation in the environment and a range of soil organic carbon adsorption coefficients from highly mobile in water to more soil bound.
To account for the effects of variable weather and environmental conditions, we ran year-long simulations between 2005 and 2020. Without information on the amount and timing of past chemical releases, we modeled hypothetical releases and resulting chemical amounts and concentrations in waterways assuming that all industrial areas started with the same potential chemical on site per unit area at the beginning of each year. The result was a range of chemical transport estimates reflecting local differences in landscape characteristics (topography, soil, imperviousness) in and around industrial facilities and differences in the volume and flow rate of receiving waterbodies.
To achieve more realistic chemical transport simulations, we customized the SWAT source code for chemical partitioning in streams. In the EDF custom version, we updated the sediment phase processes of deposition, resuspension, burial, and unburial so that they are directly coupled to modeled sediment fluxes and thus made dynamic and dependent on predicted sediment inflows, deposits and bank erosion that are a function of stream flows and conditions. Previously sorbed-phase chemical fluxes associated with these processes were constant, determined by user-provided settling and resuspension velocities. In addition, chemical buried by sediment was considered permanently removed from the stream. In EDF’s new version, buried chemical is stored and may resurface if the bed sediment in excess of the active surface sediment layer becomes resuspended in the stream. Aqueous phase processes are largely unchanged in the EDF version with the exception of a correction to the diffusion between pore water and stream water. Previously diffusion occurred proportional to the difference between total chemical mass in stream and sediment. Diffusion has been corrected to be proportional to the difference between chemical concentrations in stream water and pore water.
The modified code is open source and publicly available on Github.
The spatial domain of the SWAT model encompassed the drainage area of the San Jacinto River and other major waterways draining into Galveston Bay with two exceptions. We excluded the Trinity River basin in this model because of its smaller number of petrochemical facilities, large drainage area and outlet into a region of Galveston Bay distinct from the San Jacinto. We also excluded the drainage area around Texas City because the flat topography there made watershed delineation too uncertain given available elevation data.
Using a digital elevation model, we divided the domain into 332 subbasins, balancing the need for higher spatial resolution against computational complexity. We accomplished this by delineating larger subbasins farther upland in rural and forested headwaters and finer resolution subbasins in the dense industrial areas that were potential chemical sources. We also increased resolution at the mouth of streams entering Galveston Bay, at the sites of stream monitoring gages and at the outfalls of Addicks and Barker Reservoirs, Lake Houston and Lake Conroe.
Within each subbasin, we modeled unique combinations of dominant land use, land slope, and soil, also known as hydrologic response units (HRUs). Excluding some minor combinations limited to small areas, the model included 2,169 HRUs domain-wide.
We ran year-long SWAT simulations for 2005 to 2020 with a daily timestep using historic weather data to evaluate present-day conditions. We also ran SWAT simulations using future weather predictions from climate models during baseline (2000-2019) and future periods (2040-2059 and 2080-2099) to evaluate effects of climate change.
SWAT requires input data defining weather, elevation/topography, soil, land cover, crop and land management practices, and lakes and reservoirs.
Historic (Jan 1980-Mar 2021) daily precipitation and temperature data developed and maintained by the USDA Agricultural Research Service1 was the starting point for developing weather inputs to SWAT. We retrieved gap-filled, continuous daily data at 33 real ground-based NOAA GHCN weather stations in the study area from this dataset. From this station network, we created virtual station data at the centroid of every subbasin in the model domain by spatial interpolation using inverse-distance-weighted averages of the timeseries data at the original 33 stations. For daily evapotranspiration and solar radiation values, we ran the Weather Generator that accompanies SWAT.
Elevation data came from two sources, the more recent Upper Texas Coast 2-meter Topographic Lidar Digital Elevantion Model (DEM) LIDAR, where available in our study area (mainly Harris County), and the USGS National Map 1-arc second DEM for the rest of the domain. We merged these two datasets and resampled the elevation to a common 30 m x 30 m grid.
Soil data came from the USDA Natural Resources Conservation Service Soil Survey Geographic Database (SSURGO). Customization of SSURGO soils included merging map units with like soil parameters and renaming them by their dominant component name. The result was a new simplified soil spatial boundary dataset of major soil components.
Land cover data came from the USGS National Land Cover Database (2019). We used SWAT default values for runoff curve number and impervious surface fraction corresponding to each land cover class, except for high intensity, developed and industrial areas. We used Texas state land use codes to identify industrial land parcels that were sites for potential petrochemical releases. We parameterized these areas with higher runoff curve numbers characteristic of high impervious surface area and low vegetation.
We used SWAT default assumptions for the management and timing of crop and plant growth with the exception of perennial forests and grasses. Plant growth in SWAT affects the model’s water balance via soil water uptake and evapotranspiration, which only happen during a plant’s growth stage and cease once the plant has reached maturity. For perennial forests and grasses, we increased the plant maturity time to ensure continuous water cycling and maintain evapotranspiration.
Lakes and reservoirs in SWAT are important storage and flood control features affecting the timing and volume of water and sediment flowing through the stream network. We modeled four major reservoirs in the study area: Addicks and Barker Reservoirs, Lake Houston and Lake Conroe.
[1] White, M. J., Gambone, M., Haney, E., Arnold, J., & Gao, J. (2017). Development of a station based climate database for SWAT and APEX assessments in the US. Water (Switzerland), 9(6), 1–9. https://doi.org/10.3390/w9060437
SWAT provided daily average time series of riverine discharges of water, suspended sediment and dissolved and sorbed chemical concentrations in streams at subbasin level. SWAT outputs also included daily average time series of local yield of runoff, eroded soil and dissolved and sorbed chemical loading amounts at HRU level.
To estimate effects of climate change on toxic flooding potential, we ran simulations with future forecasts of precipitation and temperature derived from an ensemble of regionally-downscaled climate models. This data came from the Coordinated Regional Climate Downscaling Experiment (CORDEX). We chose data from the RCP8.5 scenario as a high end screening estimate from three different global climate models (GFDL-ESM, HadGEM2-ES, MPI-ESM-LR) paired with two different regional weather models (RegCM4, WRF).
With this data, we ran SWAT simulations for a 20 year baseline period (2000-2019) and two future periods mid (2040-2059) and late (2080-2099) century. Comparing the average stream flow during the baseline period to the future periods, we found a 7% increase in stream flow to Galveston Bay by mid-century and similar 6% increase by late century, associated with peak storms. There was relatively neutral change between baseline and future during non-peak times, on average.
Using this estimate for increased stream flow, we ran the coupled model for the time of hurricane Harvey (May-October, 2017), augmenting the stream flow at the boundaries of the Delft3D domains by 7% and otherwise using the same inputs for wind and tides. We did this to maintain synchronization of stormwater and storm surge as the hurricane evolved over time, resulting in a more realistic storm pattern. The resulting estimates of flood depth and duration are included as flood severity indicators in our vulnerability assessment.
- Our results are the first time, that we know about, that SWAT estimates of stream discharge and local runoff from the full Galveston Bay watershed have been used as stream boundary conditions and point sources of stormwater entering the Delft3D model domains. This allowed us to capture the combined effects of stormwater draining from farther upland in the watershed and localized storm surge on flood extent, depth and duration in dense industrial corridors.
- We used the coupled model to evaluate the benefit of community master plans that include nature-based solutions in two case studies: Galena Park, TX and Texas City, TX.
- Our modeling system included high resolution topography in holistic, nested, gridded domains that capture drainage of flood waters through small, narrow, irregular creeks and waterways.
- We ran multi-year, year-long, and multi-month simulations that account for antecedent soil moisture conditions which can have a large effect on flood incidence and magnitude.
- Our project made novel use of drogues to generate representative chemical transport pathways between flooded source facilities and destinations in distant waterways and communities.
- We created custom post-processing routines to compute flood depth and duration indicators for the vulnerability assessment.
Facility location
Uncertainty in the location of petrochemical facilities could over or underrepresent the true vulnerability of certain facilities and their potential impact on communities. Available location data through EPA’s Facility Registry Service could be limited to low quality address information such as a zip code centroid, or an office building or mailing address, and not reflective of where the actual physical facility plant is located. Large facilities may span hundreds of acres but their location is specified as just one point, typically at an entrance, often in the road making the corresponding area ambiguous. We used a variety of automated and manual approaches to geolocate the facilities more accurately and associate them with parcel areas. The large number of facilities and laborious ground truthing process means that some uncertainty still exists. We provide a locational uncertainty score for each facility in the Vulnerability map to highlight some of these issues.
Model processes
SWAT applications are typically meant to characterize long-term average and prevailing conditions based on often monthly calibrations as opposed to extreme precipitation events. Our approach to address this was a careful calibration that optimized the model performance during historic extreme events like hurricanes.
SWAT uses different equations when flow is greater than bankfull and may be less accurate than a true hydraulic model. To address this, we carefully parameterized the channel cross-sectional dimensions, paying attention to the accuracy during bankfull events in calibration. The reduced velocity of the flow when it spills into the flood plain is of particular interest for getting the timing right on the pollutant transport.
Model inputs
Topography: While 1/9 arc second CUDEM data was used where available, most of the coastal topographical information was from the 1 arc second Coastal Relief Model. The topographic resolution of this database is roughly 30 m, which may be too coarse to capture features which may play a large role in directing the flow from flooding and surge in inundated areas. In particular, features such as local depressions which can collect and pool contaminated water for some time may not be fully resolved. In addition, bathymetric information may be obsolete, particularly if the region had experienced hurricanes or other hazards after the latest bathymetric surveys. Unlike topographic elevations, which can quickly be determined from airborne LiDAR, bathymetric surveys are time-intensive, usually using rod-and-level surveys or jet skis equipped with fathometers and kinematic GPS units to measure depths along transects oriented perpendicular to shore. Nearshore effects such as wave breaking, wave-induced set-up, and surge inundation can be quite sensitive to the accuracy of nearshore bathymetry.
Local geography and the urban landscape: Any man-made features not represented in the topographical database were not incorporated into the Delft3D model. This includes streets, highways, drainage features, and sidewalks. Larger features such as the Texas City Dike and the Coastal Water Authority Canal were present in the topographic database used, and their effects were included in the modeling. In addition, infiltration of water into the soil was not included in the Delft3D model.
For SWAT, flood control features other than Addicks and Barker Reservoirs and Lake Houston and Lake Conroe were not included in the model. Industrial sites with potential chemical releases were defined with common, representative landscape parameters and assumptions for screening purposes. For evaluation of specific sites and design of nature-based solutions, models should use detailed site-specific characteristics.
Winds: While the CFSR winds are well suited to large scale investigations, they do not have the spatial resolution for local phenomena such as land-sea breezes. However, it is not anticipated that this has a significant impact on the results of this study.
Chemical transport: Representation of the transport of flood-borne contaminants with numerical drogues in Delft3D does not include any effects of dilution or evolution of contaminant concentration or toxicity.